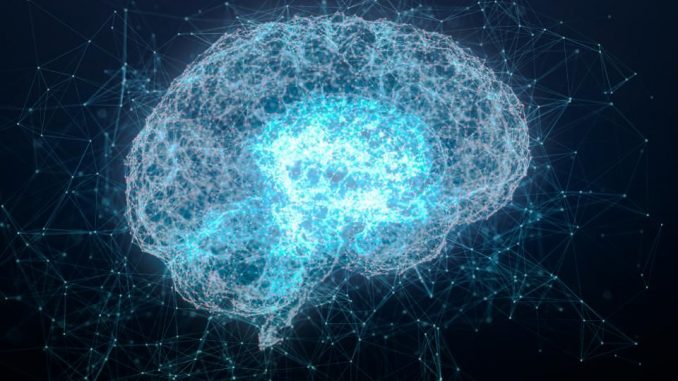
If your work puts you in regular contact with technology vendors, you’ll have heard terms such as artificial intelligence (AI), machine learning (ML), natural language processing and computer vision before. You’ll have heard that AI/ML is the future, that the boundaries of these technologies are constantly being pushed and broadened, and that AI/ML will play an integral role in shaping this tech-forward era’s most successful business models.
As a technology leader, I’ve heard all these claims and more. To say that AI/ML will play an increasingly impactful role in business is no overstatement. According to a recent Forbes article, the machine learning market is poised to more than quadruple in the coming years.
Many industry watchers agree that AI/ML solutions, when used to good effect, can equip your organization with a significant competitive advantage. And that makes it tempting to dive right in and start implementing these technologies without first gaining a comprehensive understanding of how they work. Accessibility to myriad options is not a barrier; almost every technology vendor now offers AI/ML services. If anything, we are often inundated with choices in this domain.
But how do we know we’re making the right choices and using these services to good effect? This is where a genuine, comprehensive understanding of technology becomes critically important.
For many of us, the world of AI/ML is a relatively uncharted terrain. What is artificial intelligence in modern computing? What is machine learning? The answers to these fundamental questions are the keys to unlocking the true potential of AI/ML as business solutions.
Understanding AI/ML And Its Price Of Admission
Current machine learning is a statistical process that employs a model/algorithm to explain a set of data and predict future outcomes. Many of these are “big data” algorithms that analyze huge quantities of data to generate predictions that are as accurate as possible. Once we understand this, we start to see what is required to effectively use ML as a business solution.
Simply put, we need data. We need a lot of it, and we need it to be high quality. Poor data quality is the biggest impediment to successfully adopting and deploying AI/ML solutions, and insufficient quantities of data can be a major hindrance as well.
Take IBM’s Watson for oncology as a cautionary tale. After being trained on a small number of synthetic cancer cases, the Watson supercomputer was discovered to generate “erroneous cancer treatment advice” which ranged from incorrect to outright unsafe.
The data management process, which covers everything from data creation or acquisition to transmission and storage, is therefore intrinsically linked to AI initiatives. When considering the cost of implementing any AI/ML solution, it’s vital to also consider the cost of obtaining a robust amount of high-quality data with which to feed that solution.
Considering AI/ML Solutions In The Context Of Your Needs
Now, with a better idea of what goes into deploying AI/ML solutions, we have to consider each of our options in the context of our vision. What do we hope to achieve by implementing AI/ML strategies?
Machines don’t learn in a vacuum. Any AI/ML technology we implement will function within a web of our existing applications, interfaces and platforms. So, when crafting our vision, we need to take our organization’s existing technology ecosystem into consideration.
Specificity is key in this regard. In order to choose the right model/algorithm to solve our problem, we first need to clearly define the problem we need to solve. Precise goals will help us ground our vision in reality, while a more ambiguous approach may lead to equally muddled (and unsatisfactory) results.
The Importance Of Adaptable, Unbiased Models
An effective machine learning model or algorithm must, of course, continuously learn. We won’t see much success with a “set it and forget it” mentality when it comes to machine learning algorithms. If our algorithms don’t rapidly adapt to changing requirements, they quickly become irrelevant and unproductive.
It’s just as imperative for an algorithm to be unbiased. Cathy O’Neil, the author of Weapons of Math Destruction, spoke to NPR about the dangers of placing blind faith in the objectiveness of ML algorithms when “we really have no idea what’s happening to most algorithms under the hood.”
Many of the models used today across the public and private sectors certainly suffer from the prejudices and misconceptions of their designers. In 2011, a Massachusetts man was informed his driver’s license had been revoked because a facial-recognition algorithm mistook him for another Massachusetts driver who was involved in criminal activity. In a similar vein, Google’s hate speech detector was reported to be racially biased.
The internal workings of ML algorithms are something of a black box, which makes vigilant monitoring of their predictions extremely important. To make the most of our AI/ML solutions, we have to invest the time and attention to governing them fairly and rigorously.
You might be excited, and reasonably so, about the seemingly boundless potential of AI/ML technology. Or maybe you subscribe to Stephen Hawking’s view that the development of AI could be “the worst event in the history of our civilization.”
In either case, there’s no question that AI/ML technology is here to stay. To make the most of it and avoid common pitfalls, we must keep in mind the fundamentals of AI/ML as we implement such solutions in our organizations.
source: forbes